Note to readers: we changed our name to the AI Security Institute on 14 February 2025. Read more here.
Safety cases are clear, assessable arguments that show that a system is safe in a given context. At the AI Safety Institute, we think safety cases could provide an approach for AI safety that works for both current and much higher-capability systems. Our new paper, written in collaboration with the Centre for the Governance of AI, provides a safety case template that could be used for some current AI systems.
Why safety cases?
Safety cases are used across the automotive (including autonomous vehicles), civil aviation, defence, nuclear, petrochemical, rail and healthcare industries (Favaro et al., 2023; Sujan et al., 2016; Bloomfield et al., 2012; Inge, 2007). In the case of frontier AI, two developers have highlighted safety cases as part of their approaches to safety: Google DeepMind propose to use safety cases for future systems as part of their frontier safety framework, while Anthropic noted that the recent update to their responsible scaling policy was inspired by safety case methodologies.
For safety cases to be successful in frontier AI, they need to be both practical and reliable. We don’t yet know the best way to write safety cases for frontier AI systems. We’ll need to build on best practice from other industries, while also tailoring techniques to AI’s unique circumstances.
But if we can find good ways of making these arguments, safety cases may become a robust but flexible tool assuring the safety of frontier AI systems.
Our new template shows that it’s possible to write safety cases for current systems, by bringing together existing techniques.
This work should be considered a proof of concept and focuses on a subset of relevant safety arguments. A full safety case for a current system would also include sociotechnical arguments: for example, it might argue that there is an appropriate organisational safety culture, or that relevant staff have appropriate knowledge and training. Future research from AISI will examine what else might be needed to write full safety cases, and what safety cases might look like for higher-capability systems.
Inability arguments
Our paper provides a template for making an ‘inability’ argument (Clymer et al., 2024) – that is, it argues that an AI system isn’t capable enough to pose an unacceptable level of risk. In this case, we’ve focused specifically on arguing that a system doesn’t have the necessary capabilities to increase cyber risk, but we think the approach should work well in other areas.
In effect, this inability argument is the implicit argument we make whenever we use capability evaluations to show that a system is safe. In this paper, we make the argument explicit, through the use of a safety case template. We think this has a number of advantages:
- It’s easier to spot and discuss areas of concrete disagreement. We’ve surfaced – and hopefully begun to resolve – many of these through the feedback and discussions we’ve had around this paper.
- We can more easily identify the ways in which this argument might break down for future systems.
The template: how we structure our case
We present a structure for an argument that deploying an AI system does not pose unacceptable cyber risk using Claims, Arguments, Evidence notation (Bloomfield & Netkachova, 2014).
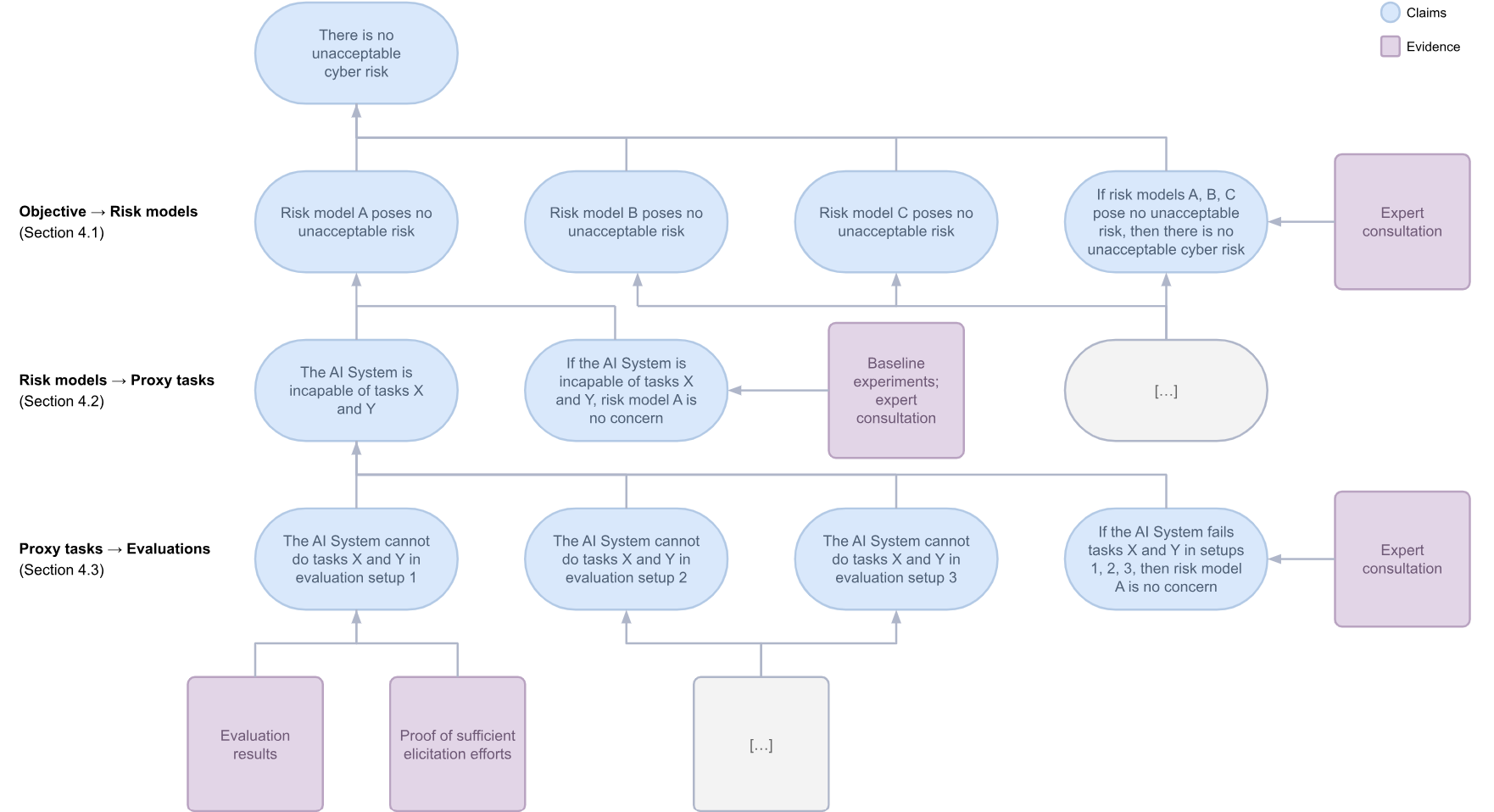
First, we break down total cyber risk into a variety of risk models. These models consist of a threat actor, a harm vector and a target (e.g. ‘the AI system uplifts a technical non-expert in vulnerability discovery and exploitation in critical national infrastructure’). We also argue that we only need to focus on the ‘easiest’ risk models – if our system is incapable of the easiest risky actions, it’s very likely to be incapable of more difficult actions.
Second, we break down these risk models into proxy capture-the-flag tasks. These tasks can be justified using expert input or baseline experiments.
Third, we determine evaluations for these proxy tasks (these could be fully automated evaluations, automated evaluations with human oversight, or human uplift experiments). These evaluations are combined with an explanation of why the evaluation is sufficient – for example, evidence that the evaluation was done in accordance with best practices to prevent sandbagging (van der Weij et al., 2024) or prosaic under-elicitation.
Is this argument sufficient?
For systems with low enough capabilities, we think this template sets out a good and indicative argument that the system isn’t capable enough to be unacceptably unsafe.
However, for higher-capability systems, this may no longer be true. As the results of capability evaluations approach thresholds past which we think that systems should be considered unsafe absent higher standards for safety and security measures being met, our confidence in this inability argument should decrease. For example, we will become increasingly uncertain about the extent to which the evaluations represent true risky capabilities, about the validity of our thresholds (in particular, whether the system could be unsafe even if below these thresholds), and about other facts about the system, such as the possibility of sandbagging. These uncertainties become more important as capabilities advance.
As a result, for higher-capability systems, we will need more complex arguments to reach a sufficient degree of confidence that a system is safe – for example, we might argue that a system has appropriate safeguards to prevent misuse. That said, we expect that these arguments will usually rely on some claims about inability. For example, an argument using safeguards to prevent misuse will need to argue that the system is not capable enough to cause harm intentionally.
AISI is working on what sorts of arguments we might need to include in safety cases for higher-capability systems. We’re looking at medium-capability safety cases, where we will need safeguards to prevent misuses as well as inability arguments. We’re also looking at high-capability safety cases, where the system itself might be sandbagging evaluations and/or actively attempting to subvert safeguards.
In this paper we don’t consider what appropriate thresholds might be. In the Seoul Ministerial Statement for advancing AI safety, innovation and inclusivity countries, including the UK, agreed to work together to the development of these thresholds. This work remains underway.
Implications for the future
This paper represents a proof of concept for safety cases. If we think that current systems are safe, largely because they are not capable enough to cause harm, then we should be able to make that argument explicit – our template makes it easier to do that. As a result, we believe it’s possible to write safety cases for current systems, and that this can be done, in part, by bringing together the evaluations work that governments and companies are already doing.
So, we’d like to see organisations begin applying templates like this one to real systems. If you are looking into doing this for a real AI system you’re developing, please do let us know. We’d be excited to work with you.